If you missed out on the previous article of this case study, please read:
3. Explore The AI Technologies Behind
In addition to the hardware of the car itself, let’s take a look at the AI technologies behind it: end-to-end solution without LIDAR, BEV+transformer, OCC network, LLM and more.
3.1 End-to-end solution without LIDAR
In fact, the vast majority of Smart Driving solutions in production use a multi-sensor fusion solution based on LiDAR and cameras.
Currently, following the steps of Tesla, with the support of Baidu Apollo, Jiyue also uses end-to-end intelligent driving functions based on perception hardware without LiDAR, which is called Point-to-Point Pilot Autopilot (PPA).
At the early stage of R&D, Jiyue developed a “vision-based + LIDAR” fusion intelligent driving program, with two independent systems supporting and complementing each other.
The two independent systems support each other and complement each other. Through LIDAR’s heterogeneous obstacle detection and better range capability, the data generated by LIDAR is continuously fed into the vision model to help the vision capability grow faster.
3.2 BEV+Transformer
Despite the standard configurations are chips and sensors in recent years, the BEV+Transformer visual perception algorithm, which uses large-scale data models to convert 2D images into 3D spatial information, has also been widely used in recent years.
Similarly, with Baidu’s Apollo, Jiyue adopts the pure vision of BEV (Bird’s Eye View) + Transformer, which connects the whole city and highway scene.
The combination of BEV and Transformer can fully utilize the spatial information of the environment provided by BEV and Transformer’s ability to model heterogeneous data from multiple sources to achieve more accurate environmental perception, longer-term motion planning, and more globalized decision-making.
To learn more about BEV + Transformer enhances perception and generalization capabilities in autonomous driving, please read:
BEV+Transformer: Next Generation of Autonomous Vehicles and Data Challenges?
The autonomous driving industry is undoubtedly one of the industries where AI technology has brought revolutionary…
3.3 OCC Network
After the realization of “pure vision” technology upgrades Occupancy Network (OCC) technology is used to replace LiDAR, because the OCC network can help Jiyue 01 reproduce the 3D scene more accurately and achieve a higher resolution.
OCC network algorithm is based on the traditional 3D target recognition capability, through the voxel way to understand and process spatial information, the scene space is divided into unitized “blocks”, which makes the visual perception without specific identification of what the object is, according to its volume, the state of movement can be judged whether there is an obstacle. In other words, it is only taken into account whether the “block” is currently occupied.
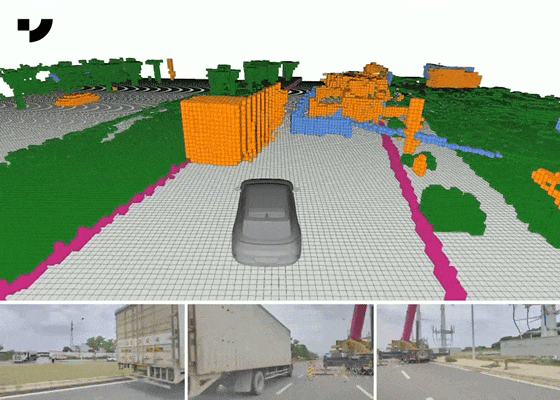
3.4 Large Language Models (LLMs) And More
The integration of Baidu’s “Ernie Bot” has given Jiyue the ability to reason, plan strategies, and generate content. The more human-computer interaction, the better it understands consumer needs.
With the rich offline voice library, users can use most of the functions of the car without any network connection, and can also conveniently use various functions in remote mountainous areas where the network is not good.
In addition, it also supports out-of-car voice interaction and in-car interaction on the layer. Includes the ability to detect sub-audible zones.
4. Dealing with massive autonomous driving data? You Need Automatic Annotation
In addition to Tesla and Jiyue, Chinese auto companies such as Xiaopeng, Huawei, Lixiang, and BYD will work on the research and development of algorithms such as BEV+Transformer, as well as the construction of an overall data closed-loop system, to gradually introduce their own urban autonomous driving functions.
Elon Musk, the founder of Tesla, also said, “In order to build Autonomy, we obviously need to train our neural net with data from millions of vehicles. This has been proven over and over again, the more training data you have, the better the results. ”
Baidu’s experiments with Jiyue also illustrate the importance of data. The data generated by LIDAR is continuously fed into the vision model to help the vision capability grow faster.
In addition, due to the complexity of roads all around the world, especially in Chinese cities, the variability of weather conditions and other factors, for the autonomous driving industry, in the face of such large and diversified labeling objects, to ensure the high quality of data labeling, and to be able to complete high-quality labeling in a relatively short period of time. It has also become an urgent problem for automated driving companies to solve.
Therefore, the quality of the data labeling directly determines the quality of the model.
Based on years of experience in the field of automated driving, maadaa.ai launched MaidX Auto-4D platform. It can significantly reduce the cost of manual annotation by 90% or more while ensuring the quality of annotation.
MaidX Auto-4D platform is a “human-in-the-loop” automatic annotation platform that supports BEV multi-sensor fusion data.
The benefits of the MaidX Auto-4D platform are:
1. Auto-Annotation Engine supporting BEV Multi-Sensor Fusion Data.
2. Support data annotation for multi-sensor fusion under BEV.
3. Omni-directional target object attributes (ID, dimension, position, classification, velocity, acceleration, occlusion relationship, visibility, trajectory, lane belonging to, etc.)
4. Forward/backward tracking is used to achieve improved detection accuracy of the current frame using historical and future information.
5. Deep learning models supporting multi-tasking (Segmentation, Object Detection, etc.)
6. Reduces manual labeling costs by 90% or more.
MaidX Auto-4D platform is a one-stop solution for efficient and high-quality annotation of massive and diversified data. While ensuring the quality, it also shortens the annotation time, reduces the annotation cost, and improves the labeling efficiency by 3 to 4 times.
For more information, please visit: