Dashcam Traffic Scenes Semantic Segmentation Dataset
Welcome to the fascinating world of autonomous driving, powered by our rich and diverse datasets. These datasets, meticulously curated and annotated, are the lifeblood of the self-driving car industry, fueling advancements across various domains.
The "Dashcam Traffic Scenes Semantic Segmentation Dataset" is essential for pushing the boundaries of autonomous driving technologies. This dataset contains driving recorder images with a resolution of about 1280 x 720 pixels, segmented semantically to reflect various elements of urban and suburban traffic environments. It comprehensively categorizes 24 different objects and scenarios, including sky, people, motor vehicles, non-motorized vehicles, highways, pedestrian paths, zebra crossings, trees, buildings, and more. This detailed semantic segmentation allows autonomous driving systems to better understand and interpret the complexities of the road, enhancing navigation and safety protocols.
Sample
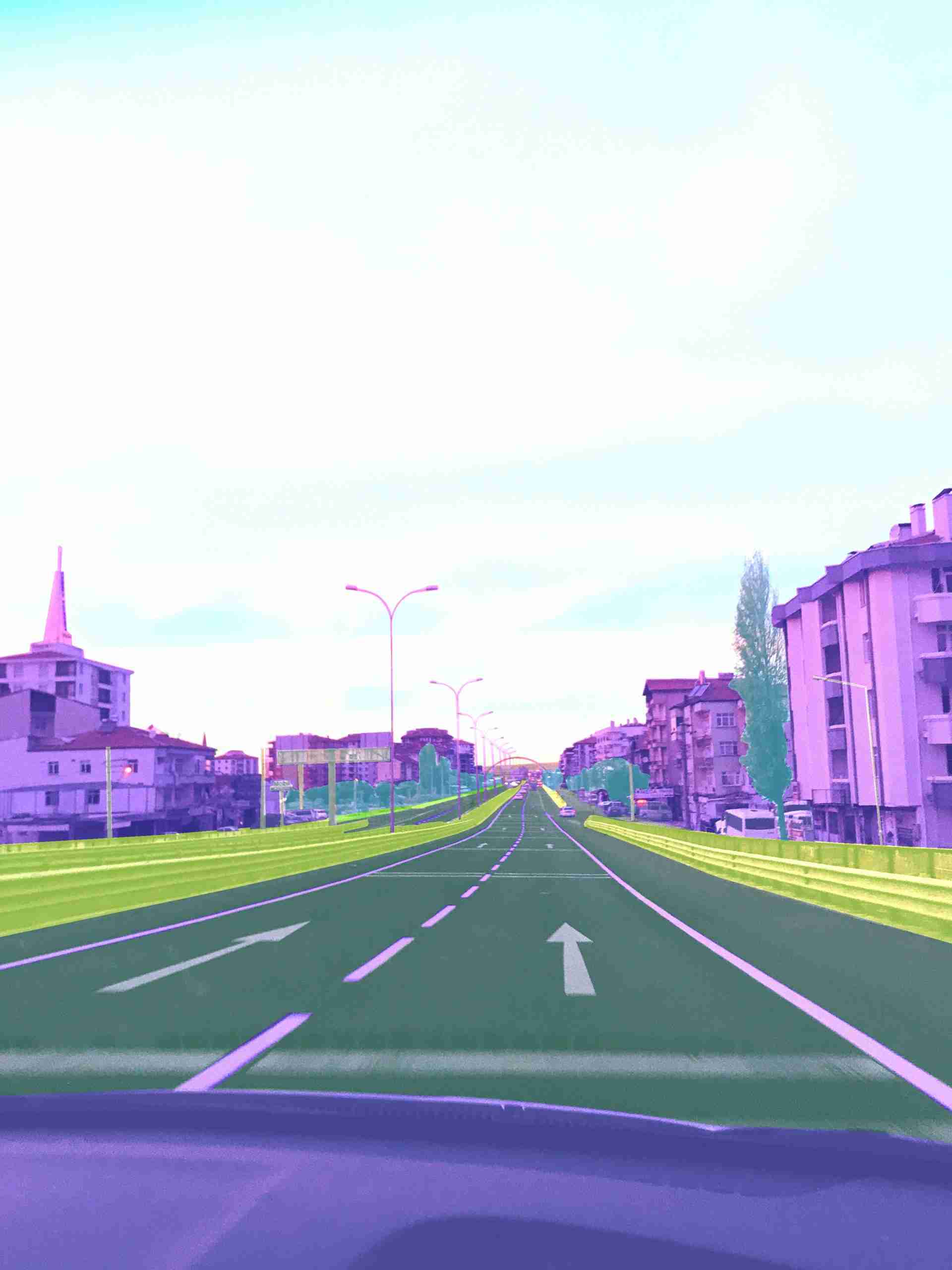
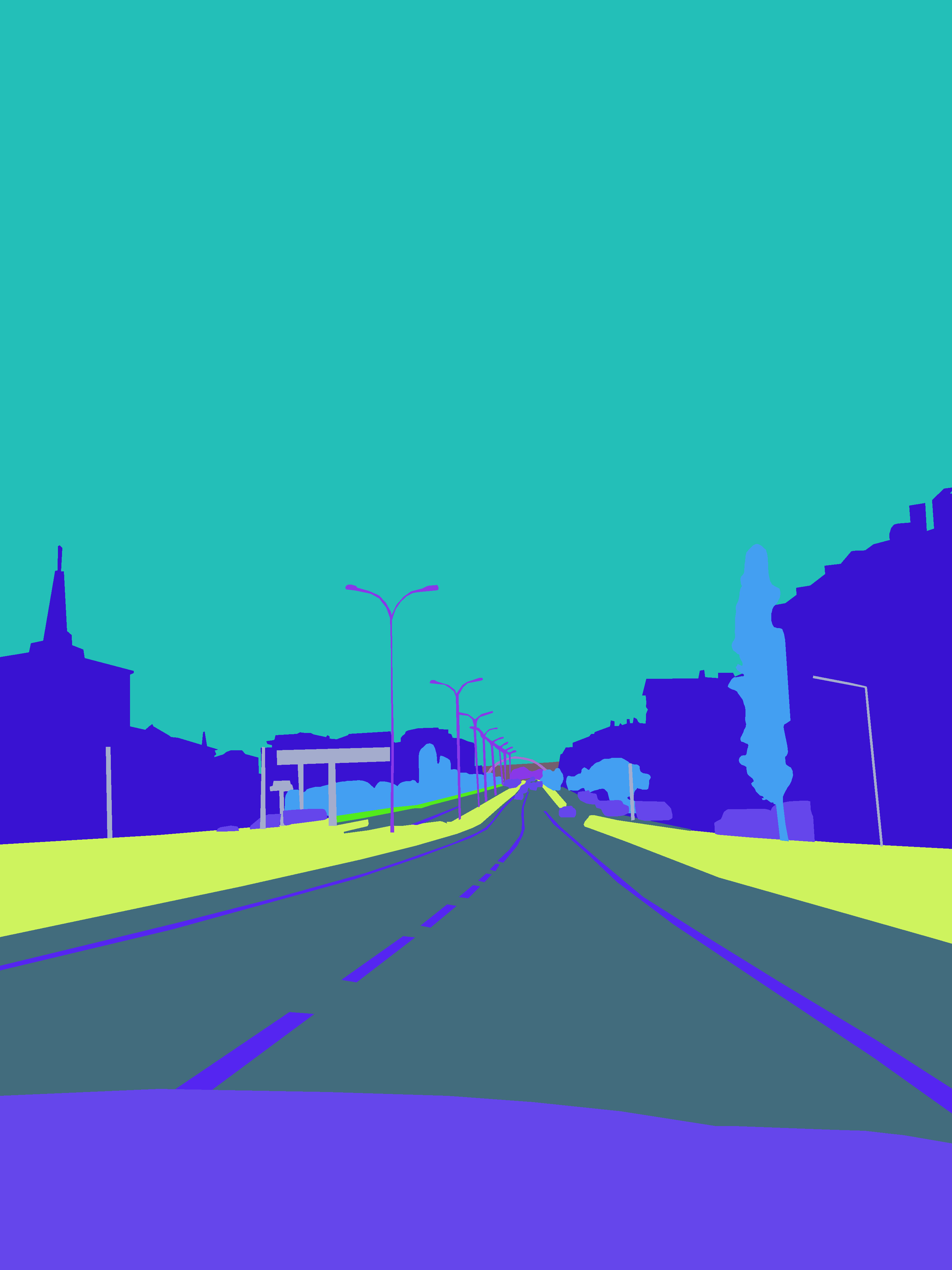
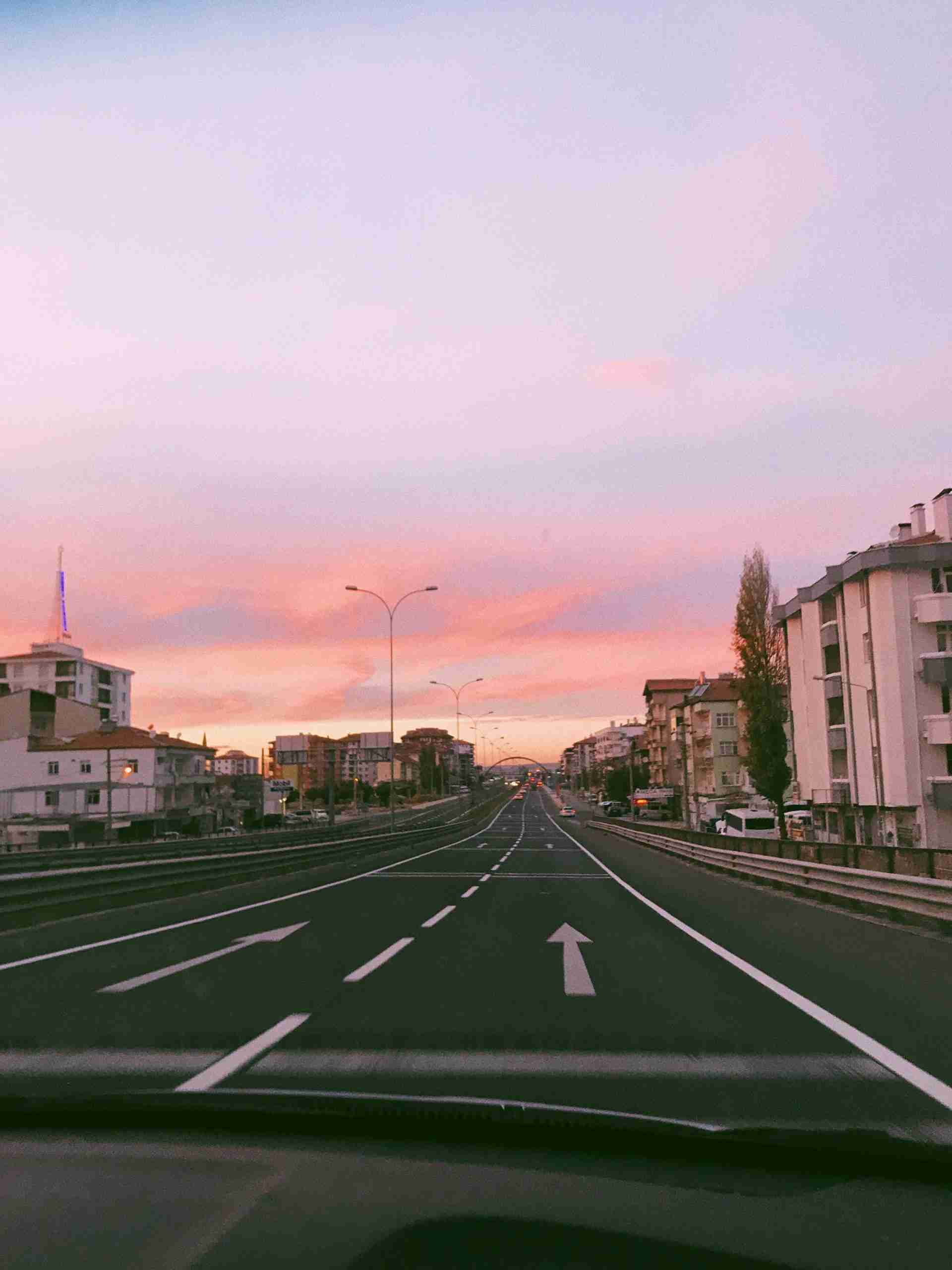
Specification
Dataset ID
MD-Auto-005
Dataset Name
Dashcam Traffic Scenes Semantic Segmentation Dataset
Data Type

Volume
About 210
Data Collection
Recording picture of driving recorder. Resolution is about 1280 x 720
Annotation
Semantic Segmentation
Annotation Notes
Including Sky, People, Motor Vehicles, Non-motorized Vehicles, Highways, Pedestrians, Other Pavement, Zebra Lines, Trees, Buildings, Columns, Bridges/Tunnels, Yellow-White Lines, Pavement Identification, Fences, Road Shoulders, Traffic Lights, Stations/Billboards, Trains, Animals, Parking Lines, Other Obstacles, Flying Objects in 24 Categories.
Application Scenarios
Autonomous Driving
Data Collections
This dataset comprises 210 high-definition images, each annotated to identify and categorize 24 different elements typically found in traffic scenes. These elements range from static objects like buildings and trees to dynamic entities such as pedestrians and vehicles, as well as road infrastructure like bridges, tunnels, and various types of pavement markings. Such a wide-ranging segmentation aids in training AI models for autonomous vehicles to accurately perceive and interact with their surroundings, ensuring safer and more efficient navigation.
Quality Assurance
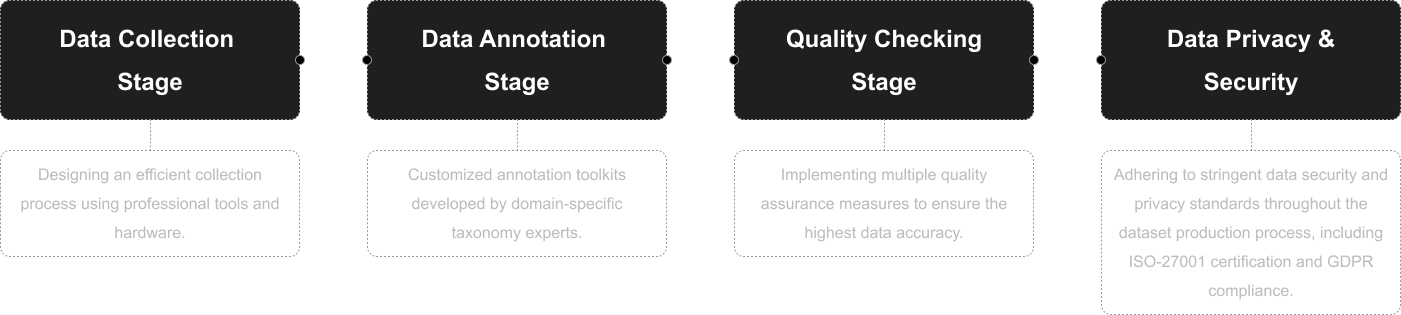
Relevant Open Datasets
To supplement our Face Parsing Dataset, users can explore these open datasets for additional resources:
Cityscapes Dataset [Learn more]
Focuses on semantic understanding of urban street scenes, featuring semantic, instance-wise, and dense pixel annotations for various classes. It includes 5,000 finely annotated images and 20,000 coarsely annotated images.
Waymo Open Dataset [Learn more]
Offers a high-quality multimodal sensor dataset for autonomous driving extracted from Waymo self-driving vehicles, covering a wide variety of environments and conditions.
nuScenes Dataset [Learn more]
A comprehensive dataset for autonomous driving that enables researchers to study urban driving situations using the full sensor suite of a real self-driving car. The dataset features camera images, lidar sweeps, and detailed map information.
A2D2 Dataset [Learn more]
The Audi Autonomous Driving Dataset (A2D2) offers a large volume of data with various annotations, including semantic segmentation and 3D bounding boxes.
Related products
Any further information, please contact us.